Study: Improvement in glucose regulation using Sugar AI app and continuous glucose monitor in adults with and without glucose disregulation
Michael Snyder, PhD; Maziyar Saberi, PhD; Tracey McLaughlin, MD
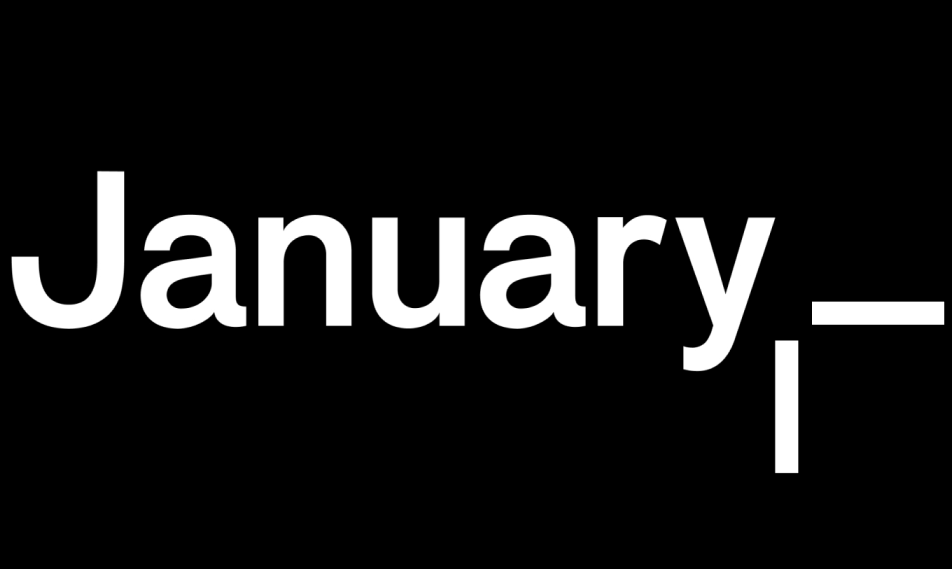
Problem Statement
The health benefits of achieving and maintaining normal blood glucose levels are well established. Continuous glucose monitors (CGM) provide a continuous measurement of glucose and offer the opportunity to track and manage glucose excursions and hypoglycemic events. The ability to monitor dramatic fluctuations in glucose offers the potential to identify and selectively modify lifestyle choices, including food choices and physical activity, that contribute to glucose dysregulation and the ensuing metabolic complications such as insulin resistance and type 2 diabetes (T2D). Improving glucose homeostasis by increasing the percent of time spent in the ideal glucose range (time-in-range; TIR) is an effective strategy to prevent or slow the progression of microvascular complications associated with glucose dysregulation and improve overall metabolic health (1, 2). While CGM use has been an effective tool for improving clinical outcomes in insulin-treated diabetes, studies examining the glucose-lowering benefits of CGM coupled with personalized lifestyle interventions in non-insulin-treated individuals are limited.
Abstract
Although CGM use has been shown to contribute to the maintenance of glucose homeostasis by decreasing both hyper- and hypoglycemia episodes in insulin-treated diabetes, its health benefits in non-insulin treated diabetes and individuals with glucose dysregulation (3) when coupled to personalized lifestyle insights is unclear. The Sugar Challenge study investigated the potential clinical benefit of CGM combined with a mobile app that links each individual’s glucose patterns to physical activity, sleep, and eating patterns in a cohort of 1022 individuals, spanning a wide range of demographic, geographic, and socioeconomic characteristics. Participants ranged from self-reported healthy (non-diabetic) to those with prediabetes and non-insulin-treated T2D. Glucose patterns were captured over ten consecutive days with CGM. Logged food intake, activities, continuous glucose, and heart rate data were captured by a smartphone-based app, Sugar AI. Sugar AI continuously provided insights to participants, overlaying daily glucose patterns with activity and food intake, including macronutrient breakdown, glycemic index (GI), and glycemic load (GL). Seventy-six percent of participants whose baseline time-in-range (TIR, 70-180 mg/dL) was <70% improved their TIR with a median of 23%. Of interest, a recent meta-analysis reported that for every absolute 10% change in %TIR, there was a 0.8% change in HbA1c (4). Analysis by subgroups showed that all three subgroups, T2D, prediabetes, and healthy showed significant improvement in TIR. These results indicate that 10-day use of CGM as a part of multimodal data collection, with synthesis and participant feedback provided by the Sugar AI app, can significantly improve glucose regulation and minimize hyperglycemia.
Background
Technology-enabled diabetes self-management approaches have gained traction as supplements or alternatives to traditional diabetes self-management models, with demonstrated improvement in HbA1c. These technologies provide a platform for the development of personalized, patient-centered interventions that integrate patient-generated health data, tailored education, and individualized feedback, which are effective behavior interventions that extend access and feasibility. Thus, new strategies that harness the benefits of technology aimed at treating and/or preventing T2D have the potential to address gaps in current approaches to lifestyle interventions and to do so at an individual level.
Continuous glucose monitoring (CGM) has emerged as a strategy to enhance glucose control in individuals diagnosed with diabetes. Studies on the use of CGM have for the most part targeted individuals on multi-dose insulin. Multiple studies in type 1 diabetes (T1D) demonstrate that the use of real-time CGM, which provides individuals with a glucose reading every 5 minutes and alarms for out-of-range glucose values, effectively reduces hyperglycemia and prevents hypoglycemia (5, 6, 7). Individuals with T2D on multi-dose insulin also show modest improvements in HbA1c (0.3%) with the use of real-time CGM as compared to self-monitoring blood glucose (SMBG) (8). Studies on T2D not on multi-dose insulin are mixed: two studies evaluating real-time CGM in 100 T2D using basal insulin, oral medications, injectable GLP-1 agonists, or a combination thereof showed improvement in HbA1c of 0.5 to 0.6% as compared to SMBG over 12-52 weeks (9, 10). By contrast, two studies in people with T2D evaluating flash-CGM (FGM), which provides glucose values for up to 8 hours after swiping a Bluetooth device over the sensor but offers no continuous data or alarms, yielded mixed results. One study (n=224) showed significantly reduced hypoglycemia but no reduction in HbA1c in those assigned to CGM vs SMBG (11), and although the second study (n=45) showed significantly improved time spent in euglycemia and reduced HbA1c compared to baseline, there was no significant benefit over SMBG (12). Only one study in non-insulin-treated T2D in Japan showed a significantly greater reduction in HbA1c (0.43%) in those assigned to flash-CGM vs SMBG (13).
Expanded use of CGM with technological aids to optimize the interpretation of glucose patterns in relationship to modifiable lifestyle factors such as food choices, eating patterns, physical activity, and sleep, has the potential to improve glycemic control in T2D as well as prevent T2D in high-risk individuals (14). To address the potential clinical utility of CGM combined with Sugar AI, we measured the change in TIR, a validated outcome measure for diabetes clinical trials (15, 16). TIR was measured in a cohort of 1022 individuals spanning a wide range of demographic, geographic, socioeconomic, and glycemic profiles who were given a flash-CGM and Sugar AI to use for 10 consecutive days (9, 2).
Results
The Sugar Challenge study was designed to examine whether a 10-day use of CGM together with Sugar AI improves TIR.
Sugar AI app enables efficient data collection

Participants were able to see their glucose patterns overlaid on their food intake and physical activity for the entire duration of the study. Participant engagement was high.
Sugar AI app engagement is the driving force for good data collection

On average, participants logged 10.7 food and activity entries per day and, a total of 25 million data points were captured across participant logs, heart rate, and continuously measured glucose data. To determine whether the use of CGM with Sugar AI improves glucose homeostasis, we compared the TIR over the last two days with those of the first two days. Over 70% of all participants in the Sugar Challenge study improved their TIR.
Absolute improvements in TIR

Among those whose baseline TIR was < 70%, 76% showed TIR improvement with a median of 23%. Moreover, 58% percent of participants who were above 180 mg/dL more than 10% of the time, improved their TIR with a median of 13%. Individuals in all classes of glucose dysregulation (T2D, prediabetes, and healthy) improved their TIR with those starting below 70% ideal TIR making the largest gains.
Individuals with T2D and prediabetes as well as those who are healthy show improvement in TIR between the first two days and last two days
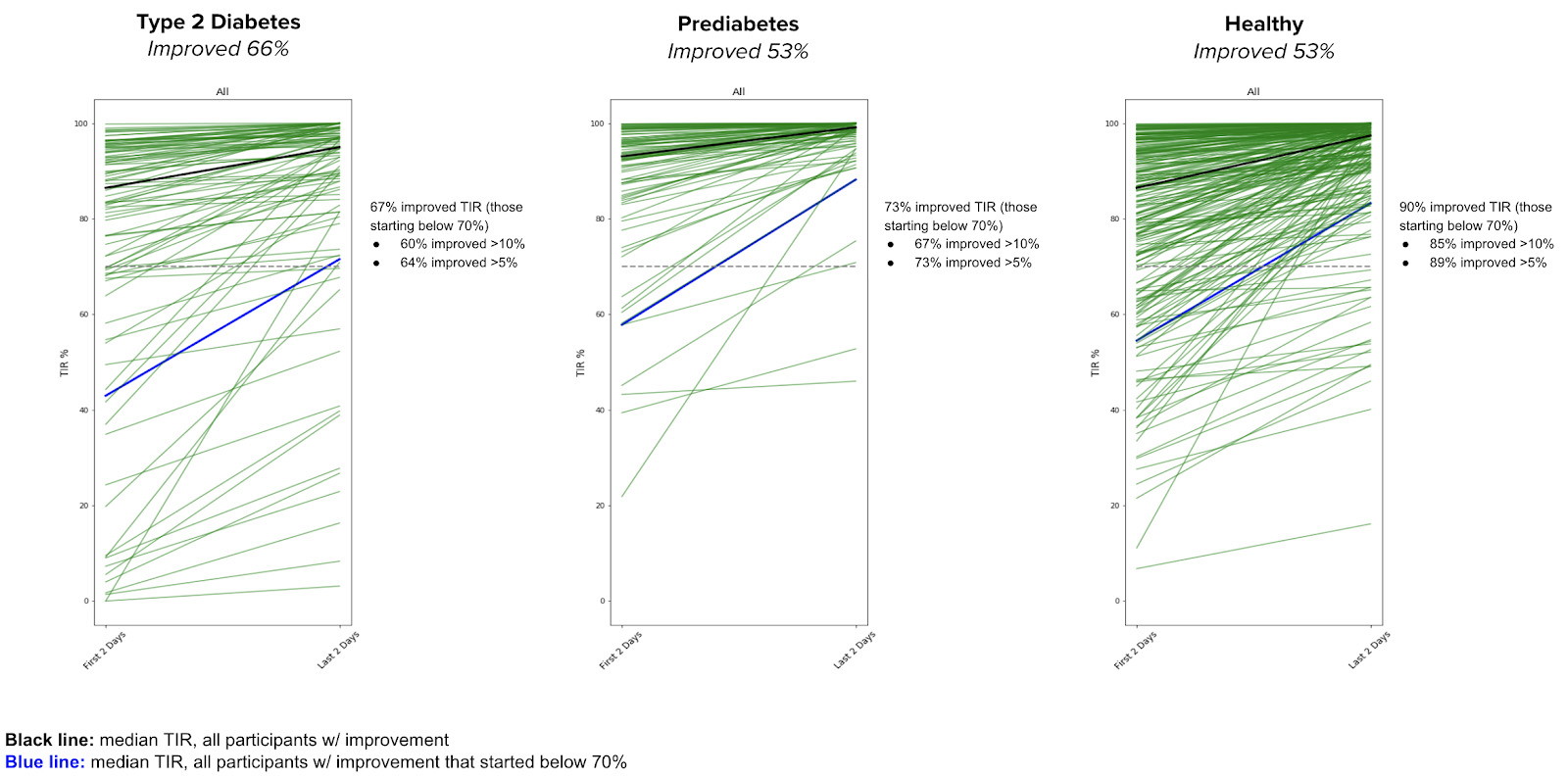
Conclusion
The use of CGM to maintain blood glucose levels in an optimal range is becoming an effective strategy not just among individuals with T1D, but also for those with T2D, prediabetes, sedentary individuals, and athletes. However, it is unclear whether the inclusion of personalized lifestyle insights such as those synthesized by Sugar AI would be of further benefit. Results from this study indicate that short-term use of CGM along with Sugar AI to guide personalized lifestyle modification can be an effective strategy to improve glucose regulation in non-insulin-treated T2D, prediabetes, and healthy individuals. Over 70% of all participants improved their TIR, and 58% of individuals with the highest blood glucose levels improved their TIR with a median of 13%. Considering the magnitude of improvement with CGM use alone, results from the Sugar Challenge study highlight the added benefit of personalized insights provided by Sugar AI. Additional studies are required to further investigate the chronic and the full benefits of Sugar AI for the management of blood glucose and overall metabolic health.
References
[1] X. Sheng, G.-H. Xiong, P.-F. Yu, and J.-P. Liu, “The Correlation between Time in Range and Diabetic Microvascular Complications Utilizing Information Management Platform,” Int. J. Endocrinol., vol. 2020, Dec. 2020, doi: 10.1155/2020/8879085.
[2] R. W. Beck et al., “Validation of Time in Range as an Outcome Measure for Diabetes Clinical Trials,” Diabetes Care, vol. 42, no. 3, pp. 400–405, Mar. 2019, doi: 10.2337/dc18-1444.
[3] H. Hall et al., “Glucotypes reveal new patterns of glucose dysregulation,” PLOS Biol., vol. 16, no. 7, p. e2005143, Jul. 2018, doi: 10.1371/journal.pbio.2005143.
[4] R. A. Vigersky and C. McMahon, “The Relationship of Hemoglobin A1C to Time-in-Range in Patients with Diabetes,” Diabetes Technol. Ther., vol. 21, no. 2, pp. 81–85, Feb. 2019, doi: 10.1089/dia.2018.0310.
[5] Juvenile Diabetes Research Foundation Continuous Glucose Monitoring Study Group et al., “Continuous glucose monitoring and intensive treatment of type 1 diabetes,” N. Engl. J. Med., vol. 359, no. 14, pp. 1464–1476, Oct. 2008, doi: 10.1056/NEJMoa0805017.
[6] T. Battelino, M. Phillip, N. Bratina, R. Nimri, P. Oskarsson, and J. Bolinder, “Effect of continuous glucose monitoring on hypoglycemia in type 1 diabetes,” Diabetes Care, vol. 34, no. 4, pp. 795–800, Apr. 2011, doi: 10.2337/dc10-1989.
[7] Juvenile Diabetes Research Foundation Continuous Glucose Monitoring Study Group et al., “Sustained benefit of continuous glucose monitoring on A1C, glucose profiles, and hypoglycemia in adults with type 1 diabetes,” Diabetes Care, vol. 32, no. 11, pp. 2047–2049, Nov. 2009, doi: 10.2337/dc09-0846.
[8] R. W. Beck et al., “Continuous Glucose Monitoring Versus Usual Care in Patients With Type 2 Diabetes Receiving Multiple Daily Insulin Injections,” Ann. Intern. Med., vol. 167, no. 6, pp. 365–374, Aug. 2017, doi: 10.7326/M16-2855.
[9] N. M. Ehrhardt, M. Chellappa, M. S. Walker, S. J. Fonda, and R. A. Vigersky, “The Effect of Real-Time Continuous Glucose Monitoring on Glycemic Control in Patients with Type 2 Diabetes Mellitus,” J. Diabetes Sci. Technol., vol. 5, no. 3, pp. 668–675, May 2011, doi: 10.1177/193229681100500320.
[10] R. A. Vigersky, S. J. Fonda, M. Chellappa, M. S. Walker, and N. M. Ehrhardt, “Short- and Long-Term Effects of Real-Time Continuous Glucose Monitoring in Patients With Type 2 Diabetes,” Diabetes Care, vol. 35, no. 1, pp. 32–38, Jan. 2012, doi: 10.2337/dc11-1438.
[11] T. Haak, H. Hanaire, R. Ajjan, N. Hermanns, J.-P. Riveline, and G. Rayman, “Flash Glucose-Sensing Technology as a Replacement for Blood Glucose Monitoring for the Management of Insulin-Treated Type 2 Diabetes: a Multicenter, Open-Label Randomized Controlled Trial,” Diabetes Ther. Res. Treat. Educ. Diabetes Relat. Disord., vol. 8, no. 1, pp. 55–73, Feb. 2017, doi: 10.1007/s13300-016-0223-6.
[12] R. A. Ajjan et al., “Sensor and software use for the glycaemic management of insulin-treated type 1 and type 2 diabetes patients,” Diab. Vasc. Dis. Res., vol. 13, no. 3, pp. 211–219, May 2016, doi: 10.1177/1479164115624680.
[13] E. Wada et al., “Flash glucose monitoring helps achieve better glycemic control than conventional self-monitoring of blood glucose in non-insulin-treated type 2 diabetes: a randomized controlled trial,” BMJ Open Diabetes Res. Care, vol. 8, no. 1, Jun. 2020, doi: 10.1136/bmjdrc-2019-001115.
[14] J. R. Petrie, A. L. Peters, R. M. Bergenstal, R. W. Holl, G. A. Fleming, and L. Heinemann, “Improving the clinical value and utility of CGM systems: issues and recommendations : A joint statement of the European Association for the Study of Diabetes and the American Diabetes Association Diabetes Technology Working Group,” Diabetologia, vol. 60, no. 12, pp. 2319–2328, Dec. 2017, doi: 10.1007/s00125-017-4463-4.
[15] I. B. Hirsch, J. L. Sherr, and K. K. Hood, “Connecting the Dots: Validation of Time in Range Metrics With Microvascular Outcomes,” Diabetes Care, vol. 42, no. 3, pp. 345–348, Mar. 2019, doi: 10.2337/dci18-0040.
[16] M. A. L. Gabbay et al., “Time in range: a new parameter to evaluate blood glucose control in patients with diabetes,” Diabetol. Metab. Syndr., vol. 12, no. 1, p. 22, Mar. 2020, doi: 10.1186/s13098-020-00529-z.